Protein-Protein Interactions
Identification of protein-protein interactions (PPIs) is important to elucidate protein functions and research biological processes in a cell. The knowledge of PPIs can help people understand disease mechanism, and suggest many technologies for designing drugs. In past several years, a large number of methods have been developed for the large-scale analysis of PPIs. It is possible to accurately predict PPIs only using protein sequence information. In order to obtain good prediction performance, the most important computational challenge is to effectively represent a protein sequence by a fixed length feature vector.
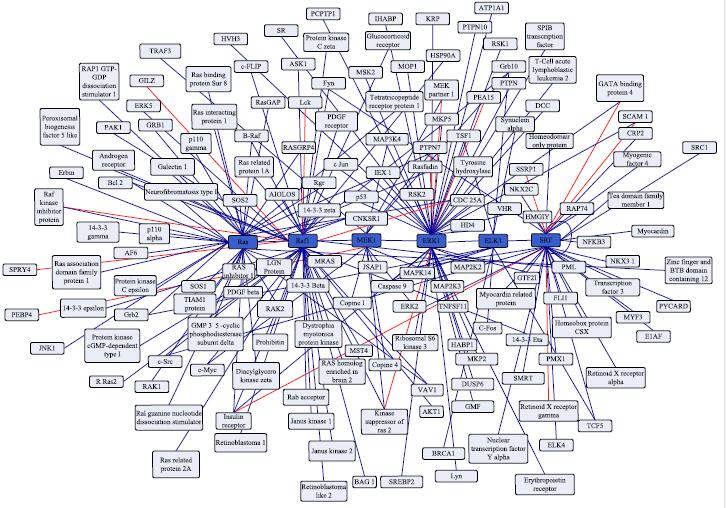
Drug–Target Interactions
Identification of drug–target interactions (DTIs) is an important process in drug discovery. The computational models have been developed to predict potential drug–target associations on a large scale. Among them, the machine learning-based methods could efficiently large-scale analysis DTIs. The DTIs is a bipartite network. Therefore, identification of new associations between drugs and targets is a recommender task. The Regularized Least Square (RLS), graph Laplacian regularized, Matrix Factorization (MF), Deep learning and Multiple Kernel Learning (MKL) et al. have been widely used in identification of DTIs.
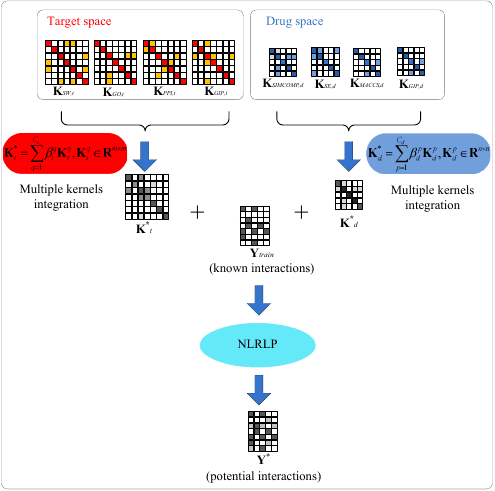
ncRNA-Disease Associations
Identifying accurate associations between non coding RNAs (ncRNA) and diseases is beneficial for diagnosis and treatment of human diseases. Traditional verifcation methods for ncRNA-disease associations take a lot of time and expense, so it is especially important to design computational methods for detecting potential associations. Most of computational methods are based on the assumption that ncRNAs with high similarity apt to be related with similar diseases and vice versa. Considering the restrictions of previous computational methods for predicting potential ncRNAs-disease associations, we develop some framework to break through the limitations. First, we extract mutiple ncRNA similarity kernels and mutiple disease similarity kernels. Then, we combine these kernels into a single kernel through the multiple kernel learning model, and use some methods to eliminate noise in the similarity kernel. Finally, we find candidate associations via machine learning.
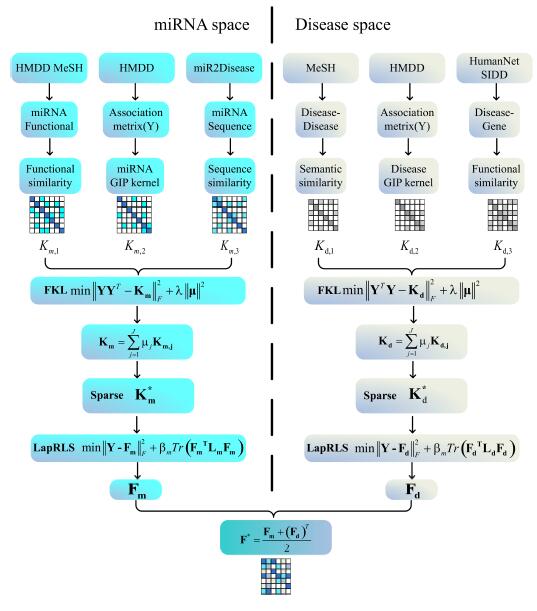
Protein Subcellular Localization
Identifying the location of proteins in a cell plays an important role in understanding their functions, such as drug design, therapeutic target discovery and biological research. However, the traditional subcellular localization experiments are time-consuming, laborious and small scale. With the development of next generation sequencing technology, the number of proteins has grown exponentially, which lays the foundation of the computational method for identifying protein subcellular localization. Multi-label protein localization is becoming more and more important.
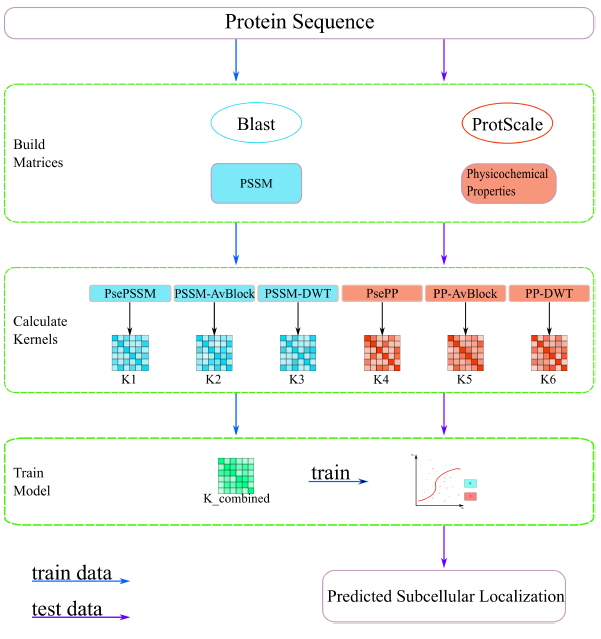